Unveiling the Power of Markov Chain Aggregation for Agent-Based Models: A Comprehensive Guide

In the realm of complexity theory, agent-based models (ABMs) emerge as invaluable tools for simulating and understanding intricate systems. These models capture the dynamic interactions of individual agents, allowing researchers to delve into the emergent properties and collective dynamics of complex systems. However, the sheer volume of data generated by ABMs often presents challenges when it comes to analyzing and interpreting the results effectively.
This is where Markov chain aggregation (MCA) steps in as a powerful technique that addresses this issue. MCA offers a systematic approach to reduce the computational complexity of ABMs while preserving key information essential for understanding the system's behavior.
5 out of 5
Language | : | English |
File size | : | 6944 KB |
Text-to-Speech | : | Enabled |
Enhanced typesetting | : | Enabled |
Word Wise | : | Enabled |
Print length | : | 209 pages |
Screen Reader | : | Supported |
Paperback | : | 192 pages |
Item Weight | : | 1 pounds |
Dimensions | : | 6.1 x 9.25 inches |
Hardcover | : | 113 pages |
X-Ray for textbooks | : | Enabled |
Markov Chains: A Brief Overview
Before exploring MCA, it is crucial to understand the fundamental concept of Markov chains. A Markov chain is a stochastic process that describes a sequence of states where the probability of transitioning from one state to another depends solely on the current state. This property is known as the Markov property.
$$ P(X_{t+1}=x_{t+1}| X_{t}=x_{t}, X_{t-1}=x_{t-1}, ..., X_0=x_0) = P(X_{t+1}=x_{t+1}| X_{t}=x_{t}) $$
In other words, the future evolution of a Markov chain is independent of its past history, given the current state. This characteristic makes Markov chains a useful tool for modeling systems with short-term memory.
Markov Chain Aggregation: Bridging the Gap in ABMs
Markov chain aggregation builds upon the Markov chain concept to address the complexity of ABM simulations. The technique involves aggregating individual agent states into a smaller set of collective states, known as metastates. These metastates represent the distribution of agent states at a higher level of abstraction.
The key to MCA lies in the assumption that the aggregated system behaves as a Markov chain. This is achieved by ensuring that the transition probabilities between metastates are independent of the specific agent configurations within each metastate.
Benefits of Markov Chain Aggregation
The benefits of Markov chain aggregation for agent-based models are multifaceted:
1. Reduced Computational Complexity: By reducing the number of states, MCA significantly decreases the computational burden of ABM simulations. This enables researchers to explore larger and more complex systems that would otherwise be intractable.
2. Enhanced Interpretability: Aggregated metastates offer a simplified representation of the system, making it easier to understand the relationships between different components and identify dominant patterns.
3. Improved Prediction: MCA helps identify metastable states, which represent stable configurations of the system. This knowledge can be leveraged to predict future behavior and anticipate system transitions.
4. Efficient Calibration: The reduced dimensionality of the aggregated system facilitates the calibration of ABM parameters, making it less time-consuming and resource-intensive.
Applications of Markov Chain Aggregation
The applicability of Markov chain aggregation extends across various scientific disciplines and complex systems, including:
1. Social Dynamics: Understanding the emergence of social norms and the diffusion of ideas in social networks.
2. Epidemiological Modeling: Simulating the spread of infectious diseases and evaluating the effectiveness of control measures.
3. Ecological Systems: Investigating the dynamics of predator-prey interactions and the impact of environmental factors on species populations.
4. Economic Systems: Modeling the behavior of financial markets and analyzing the interactions between economic agents.
5. Traffic Management: Optimizing traffic flow and understanding the dynamics of congestion in urban transportation systems.
Markov chain aggregation has emerged as a powerful technique in the field of agent-based modeling. By reducing computational complexity, enhancing interpretability, and providing predictive insights, MCA empowers researchers to tackle complex systems that were previously inaccessible. As the complexity of real-world phenomena continues to grow, MCA is poised to play an increasingly vital role in advancing our understanding of these systems and shaping future scientific discoveries.
Whether you are a researcher in the field of complexity science, a student seeking to delve into the realm of ABMs, or simply an individual fascinated by the intricate workings of complex systems, the book "Markov Chain Aggregation For Agent Based Models Understanding Complex Systems" offers a comprehensive guide to this groundbreaking technique. With its in-depth explanations, illustrative examples, and cutting-edge research, this book will equip you with the knowledge and skills to unlock the full potential of Markov chain aggregation and unravel the mysteries of complex systems.
Embrace the power of Markov chain aggregation and embark on a journey of discovery, where the intricacies of complex systems yield to your understanding. The future of scientific exploration lies in embracing sophisticated techniques that bridge the gap between computational feasibility and scientific insight.
5 out of 5
Language | : | English |
File size | : | 6944 KB |
Text-to-Speech | : | Enabled |
Enhanced typesetting | : | Enabled |
Word Wise | : | Enabled |
Print length | : | 209 pages |
Screen Reader | : | Supported |
Paperback | : | 192 pages |
Item Weight | : | 1 pounds |
Dimensions | : | 6.1 x 9.25 inches |
Hardcover | : | 113 pages |
X-Ray for textbooks | : | Enabled |
Do you want to contribute by writing guest posts on this blog?
Please contact us and send us a resume of previous articles that you have written.
Book
Novel
Page
Chapter
Text
Story
Genre
Reader
Library
Paperback
E-book
Magazine
Newspaper
Paragraph
Sentence
Bookmark
Shelf
Glossary
Bibliography
Foreword
Preface
Synopsis
Annotation
Footnote
Manuscript
Scroll
Codex
Tome
Bestseller
Classics
Library card
Narrative
Biography
Autobiography
Memoir
Reference
Encyclopedia
Diogenes Ruiz
Tom Olivadotti
Devangini Patel
Donald J Bingle
Fabio Bagarello
Joe Ducie
Don Kesterson
Wendy Lebolt
Nick Garbutt
Kai S Lam
Nicholas Ind
Don Lessem
Thomas Molnar
Derek Landy
Denise Deegan
Mel Corrigan
Donald Margulies
David Phillips
Dellables Inc
Dennis G Zill
Light bulbAdvertise smarter! Our strategic ad space ensures maximum exposure. Reserve your spot today!
- Caleb LongFollow ·10.9k
- Charles DickensFollow ·19k
- Gilbert CoxFollow ·9.4k
- Geoffrey BlairFollow ·11k
- Tyrone PowellFollow ·6.9k
- Jorge Luis BorgesFollow ·13.4k
- Henry GreenFollow ·10.4k
- Allen ParkerFollow ·8.8k


Unveiling the Return of the Antichrist and the New World...
As darkness descends upon the...


Embark on an Unforgettable Journey: "Something Lost...
Prepare to be captivated as you...
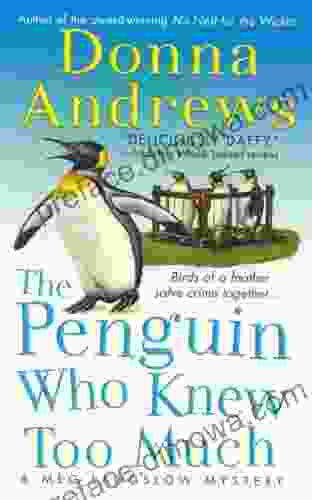

"The Penguin Who Knew Too Much": A Captivating Mystery on...
Prepare yourself for an...
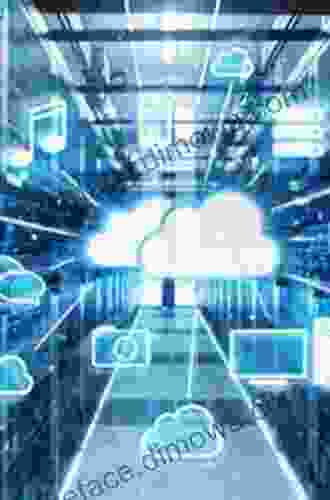

Master the Art of Staying Present and Profitable in the...
In the relentless...


Escape into a Tropical Paradise with "Toucan Keep Secret"
Immerse Yourself in the Vibrant World of a...
5 out of 5
Language | : | English |
File size | : | 6944 KB |
Text-to-Speech | : | Enabled |
Enhanced typesetting | : | Enabled |
Word Wise | : | Enabled |
Print length | : | 209 pages |
Screen Reader | : | Supported |
Paperback | : | 192 pages |
Item Weight | : | 1 pounds |
Dimensions | : | 6.1 x 9.25 inches |
Hardcover | : | 113 pages |
X-Ray for textbooks | : | Enabled |